What Kind of AI Infrastructure is Best for my Business?
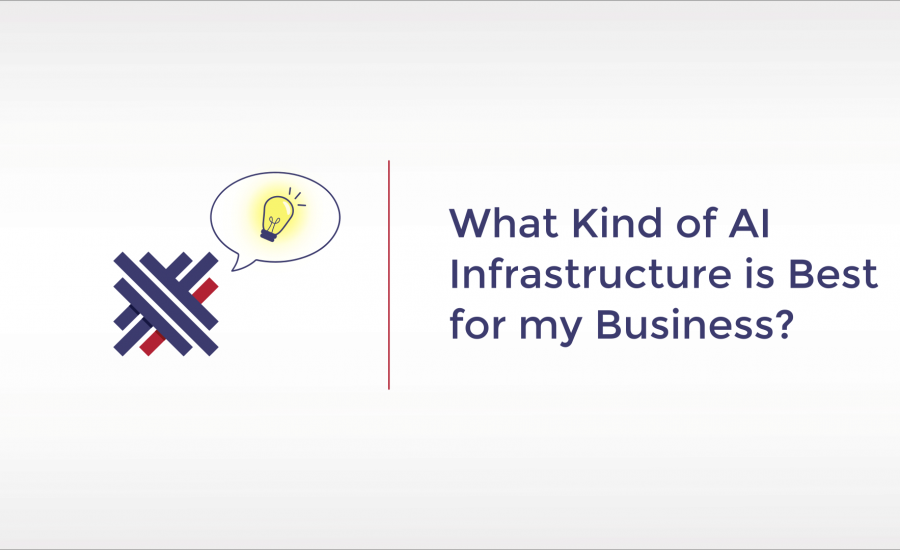
In this week’s Exponential Chats, some of the team members responsible for Amalgam’s development will have a chat about the various infrastructure alternatives available when it comes to training and deployment of Artificial Intelligence models. Between Cloud, Colocation, and On-Premise which one would you say is the best infrastructure for your AI needs? Come join us and participate by asking questions or giving your opinion in the live chat.
– Adriano Marques is the founder and CEO of Exponential Ventures.
– Nathan Martins is a Machine Learning/DevOps Engineer at Exponential Ventures, where he works on projects to democratize AI, as well as other cutting edge innovations.
– Rhuan Silva works as FullStack, DevOps Engineer and now he’s majoring in Big Data and Artificial Intelligence.
– Fernando Camargo is a Data Scientist with in-depth machine learning expertise and wide-ranging experience in software engineering.
Creating Artificial Intelligence often requires a lot of computing resources. This is especially true when dealing with most Machine Learning techniques. When starting out, most Machine Learning engineers quickly learn how inadequate laptops and desktops are when it comes to serving as a platform for the development of AI models. In fact, the requirements are so extreme that even your typical server-grade hardware is inept for the job. That is why Engineers have been resorting to the big guns, such as GPUs, ultra-high-speed networks, and highly parallelized distributed storage solutions. All of this comes with a price tag, and I’ve got to tell you… it is not cheap.
There are three types of infrastructure to pick from: the Cloud, colocation, and on-premise.
The Cloud has become the preferred type of infrastructure for essentially any kind of business in need of hardware. The three major cloud providers are Amazon, Google, and Microsoft. Part of the appeal is the fact that you’re not required to acquire any physical hardware or supporting infrastructure, and you pay only for resources that you use. This is great for most businesses because it allows for instantaneous access to hardware and infrastructure without having to figure out how to acquire and set these up. This kind of infrastructure has facilitated an uncountable number of startups and apps that benefit us on a daily basis. It is great for almost everything, but when it comes to Artificial Intelligence, it fails dramatically in two important aspects: performance and price. Because all hardware and infrastructure are shared between different customers, you’re often unable to achieve the desired performance with the hardware you’re renting and when you do, you’re probably impacting the performance of someone else. And finally, there is the price problem. While startups are able to rent servers for as cheap as $0.002 per hour, when committing to full usage for 3 years, when it comes to hardware capable of supporting any kind of AI workload, you’ll be paying $9.057 per hour on a single server, again, when committing to full usage for 3 years. That comes up to a bill of $241,278 at the end of the 3 years with a single server and not counting the other charges related to data transfers. For that reason, Cloud infrastructure has been prohibitively expensive to most companies. Even big ones! Take it from NASA: they forgot to account for the cost of data transfers and ended up with the prospect of having to pay an extra $30 million dollars to their yearly budget if they wanted to do anything with the machines they reserved with AWS.
Colocation solves some, but not all of these problems. It gets most of the costly infrastructure issues out of the way, such as cooling and power redundancy, but leaves you having to acquire and manage the hardware on your own. Plus, access to the facility is not easy due to the extraneous access control measures, and finally, you end up sharing some resources with hundreds of other companies in the same datacenter, which can impact your business in different ways. It is also very expensive: the monthly dues for a single rack are on average $2,500 discounting other fees that add up based on the kind of access and add-ons you’re looking to benefit from.
Finally, there is On-Premise. It is admittedly, the hardest one to manage and with the highest upfront cost. But when it comes to AI it is the CHEAPEST in the long run, the one that affords the most freedom, and the most performant. Since you won’t be sharing resources with anyone else, you’re free to experiment and reach the capacity of your hardware without fear of racking up fees or enduring throttling. Moreover, you’re in complete control of your hardware and how you want to support it.
Despite the cost and performance discrepancies, I believe that different businesses will benefit more from different solutions. Which is why we’re having this discussion today.
Exponential Chats is a live event conducted by our parent company, Exponential Ventures. In this event, our team members and guests have an in-depth conversation about Exponential Technologies, Entrepreneurship, and some of the world’s most challenging outstanding problems.
This live episode streamed on Thrusday, October 1st 2020 at 1:00 PM CST. Here is a link to video on Youtube: https://www.youtube.com/watch?v=h0Ap0VP0rgE
THE BLOG
News, lessons, and content from our companies and projects.
41% of small businesses that employ people are operated by women.
We’ve been talking to several startups in the past two weeks! This is a curated list of the top 5 based on the analysis made by our models using the data we collected. This is as fresh as ...
Porto Seguro Challenge – 2nd Place Solution
We are pleased to announce that we got second place in the Porto Seguro Challenge, a competition organized by the largest insurance company in Brazil. Porto Seguro challenged us to build an ...
Predicting Reading Level of Texts – A Kaggle NLP Competition
Introduction: One of the main fields of AI is Natural Language Processing and its applications in the real world. Here on Amalgam.ai we are building different models to solve some of the problems ...
Porto Seguro Challenge
Introduction: In the modern world the competition for marketing space is fierce, nowadays every company that wants the slight advantage needs AI to select the best customers and increase the ROI ...
Sales Development Representative
At Exponential Ventures, we’re working to solve big problems with exponential technologies such as Artificial Intelligence, Quantum Computing, Digital Fabrication, Human-Machine ...
Exponential Hiring Process
The hiring process is a fundamental part of any company, it is the first contact of the professional with the culture and a great display of how things work internally. At Exponential Ventures it ...
Exponential Ventures annonce l’acquisition de PyJobs, FrontJobs et RecrutaDev
Fondé en 2017, PyJobs est devenu l’un des sites d’emploi les plus populaires du Brésil pour la communauté Python. Malgré sa croissance agressive au cours de la dernière année, ...
Exponential Ventures announces the acquisition of PyJobs, FrontJobs, and RecrutaDev
Founded in 2017, PyJobs has become one of Brazil’s most popular job boards for the Python community. Despite its aggressive growth in the past year, PyJobs retained its community-oriented ...
Sales Executive
At Exponential Ventures, we’re working to solve big problems with exponential technologies such as Artificial Intelligence, Quantum Computing, Digital Fabrication, Human-Machine ...
What is a Startup Studio?
Spoiler: it is NOT an Incubator or Accelerator I have probably interviewed a few hundred professionals in my career as an Entrepreneur. After breaking the ice, one of the first things I do is ask ...
Social Media
At Exponential Ventures, we’re working to solve big problems with exponential technologies such as Artificial Intelligence, Quantum Computing, Digital Fabrication, Human-Machine ...
Hunting for Unicorns
Everybody loves unicorns, right? But perhaps no one loves them more than tech companies. When hiring for a professional, we have an ideal vision of who we are looking for. A professional with X ...
Stay In The Loop!
Receive updates and news about XNV and our child companies. Don't worry, we don't SPAM. Ever.